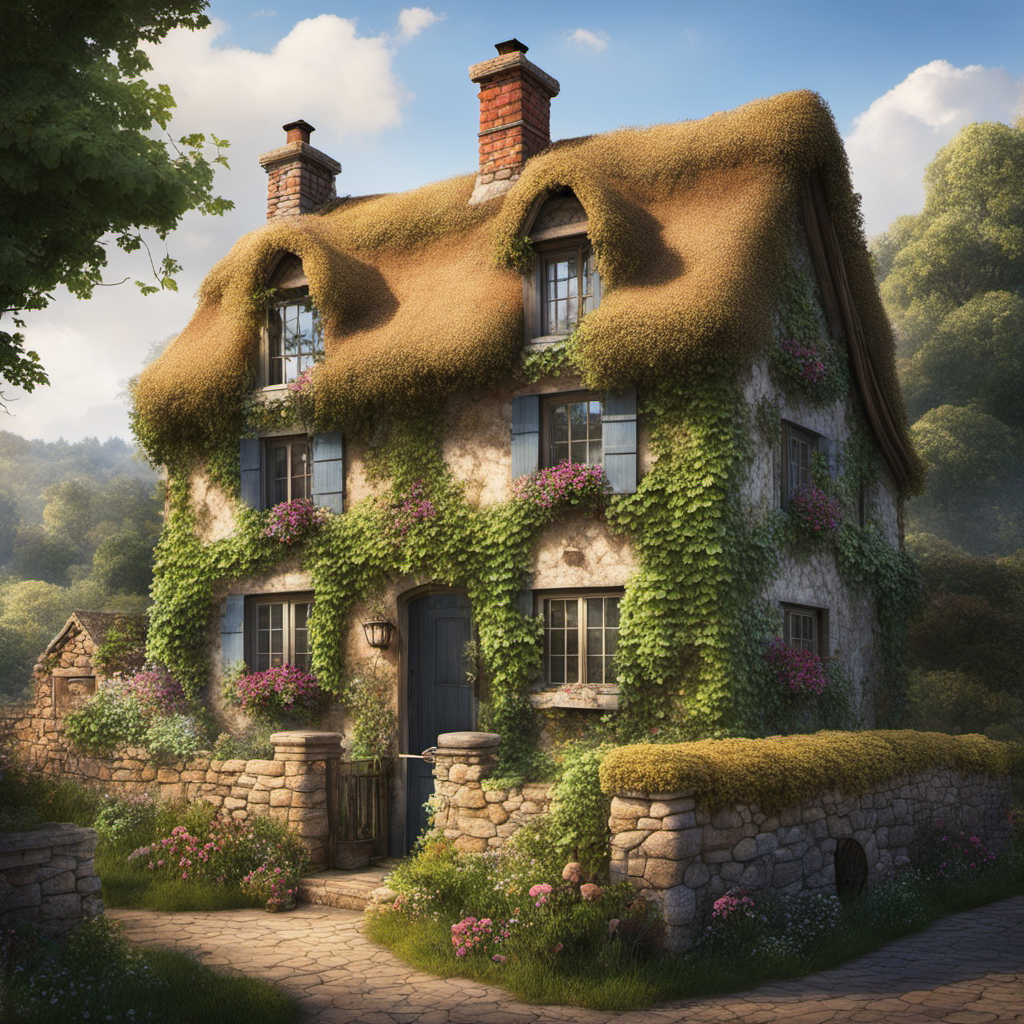
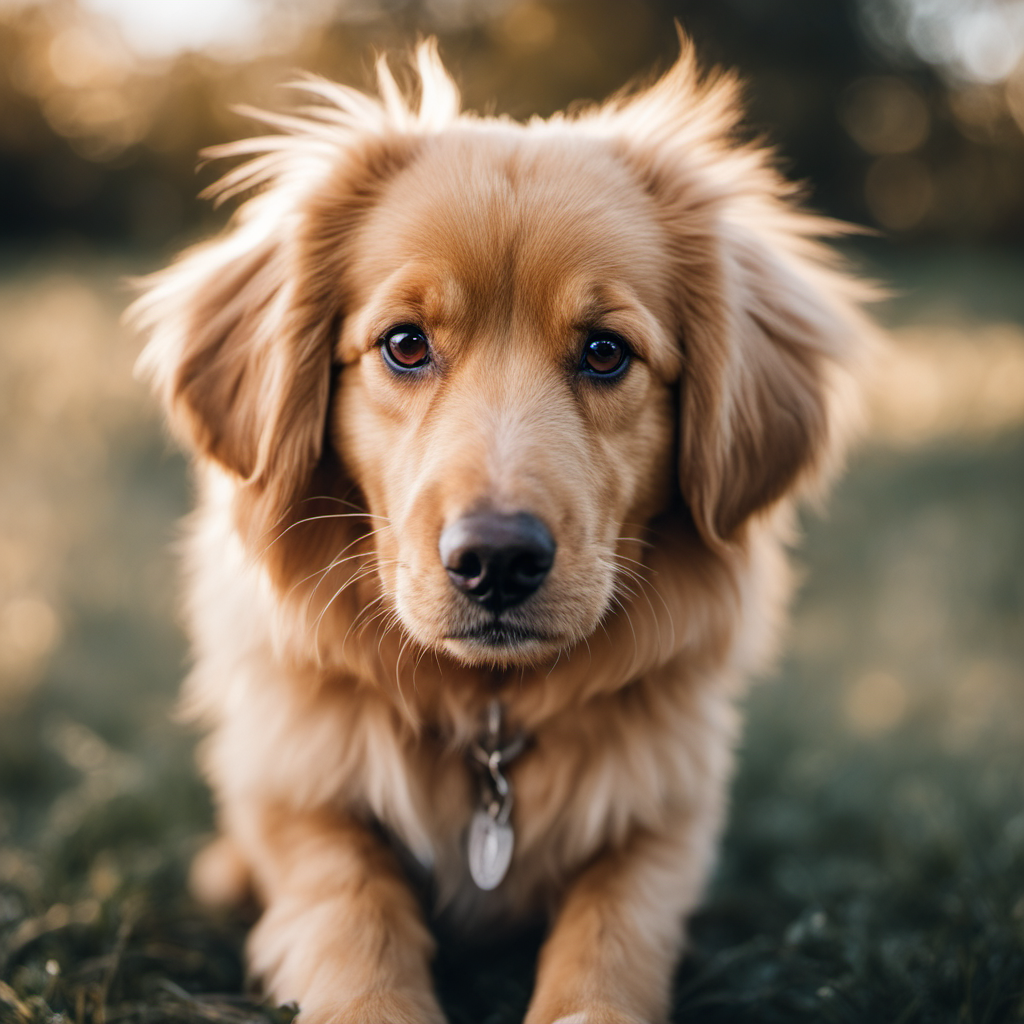
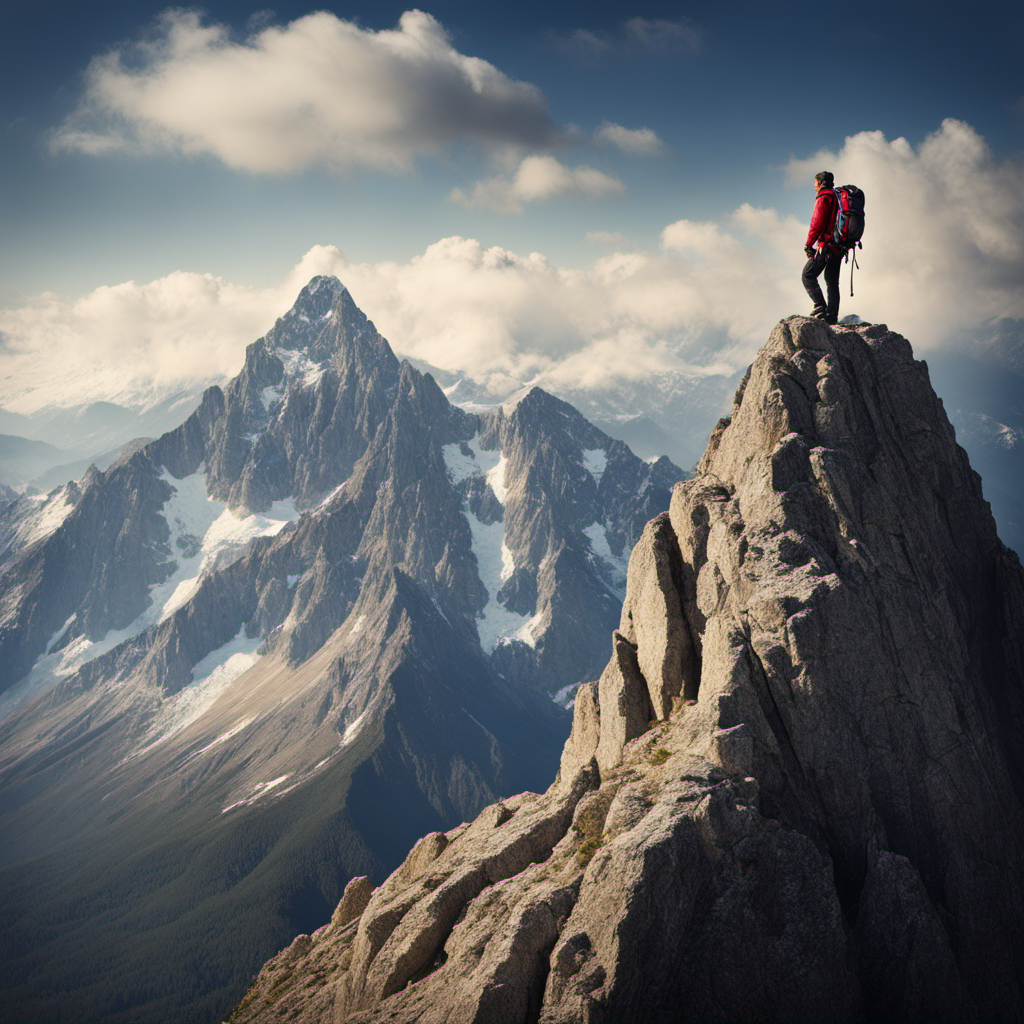
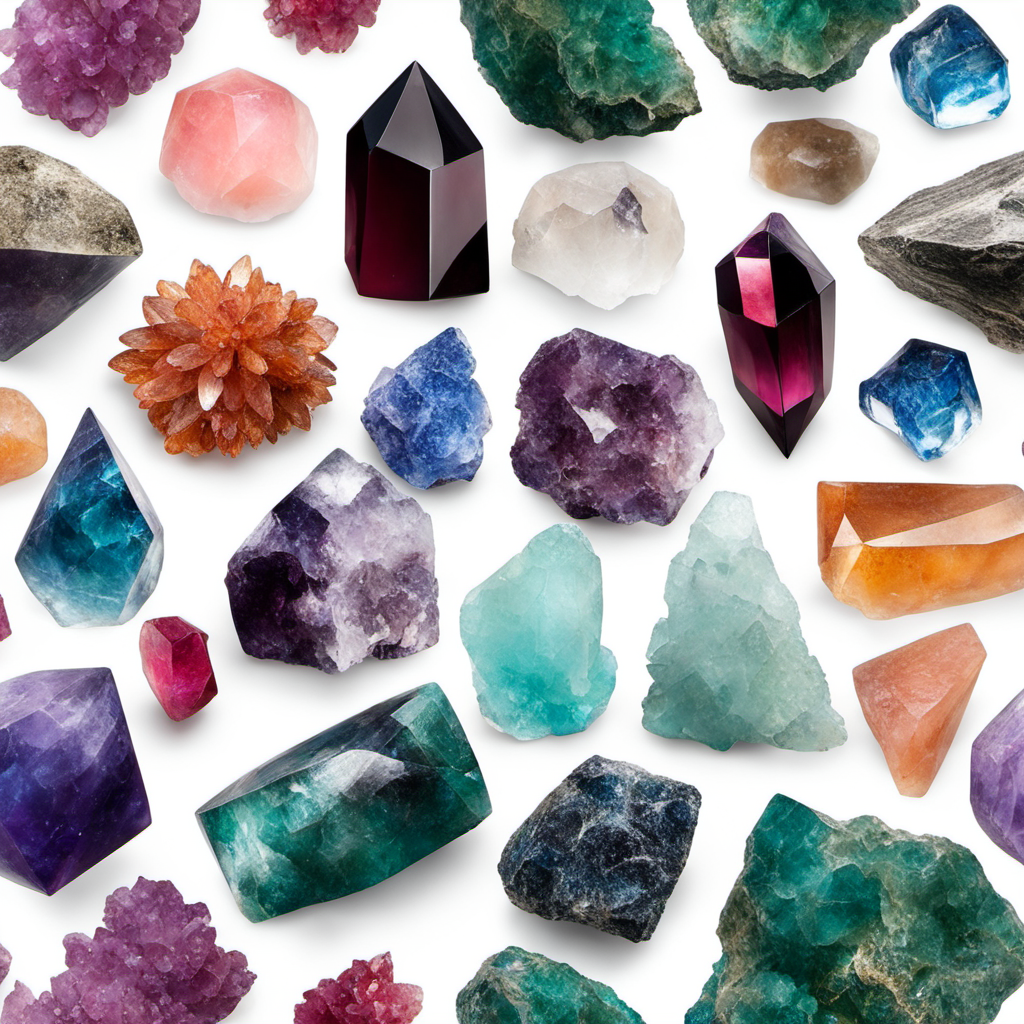
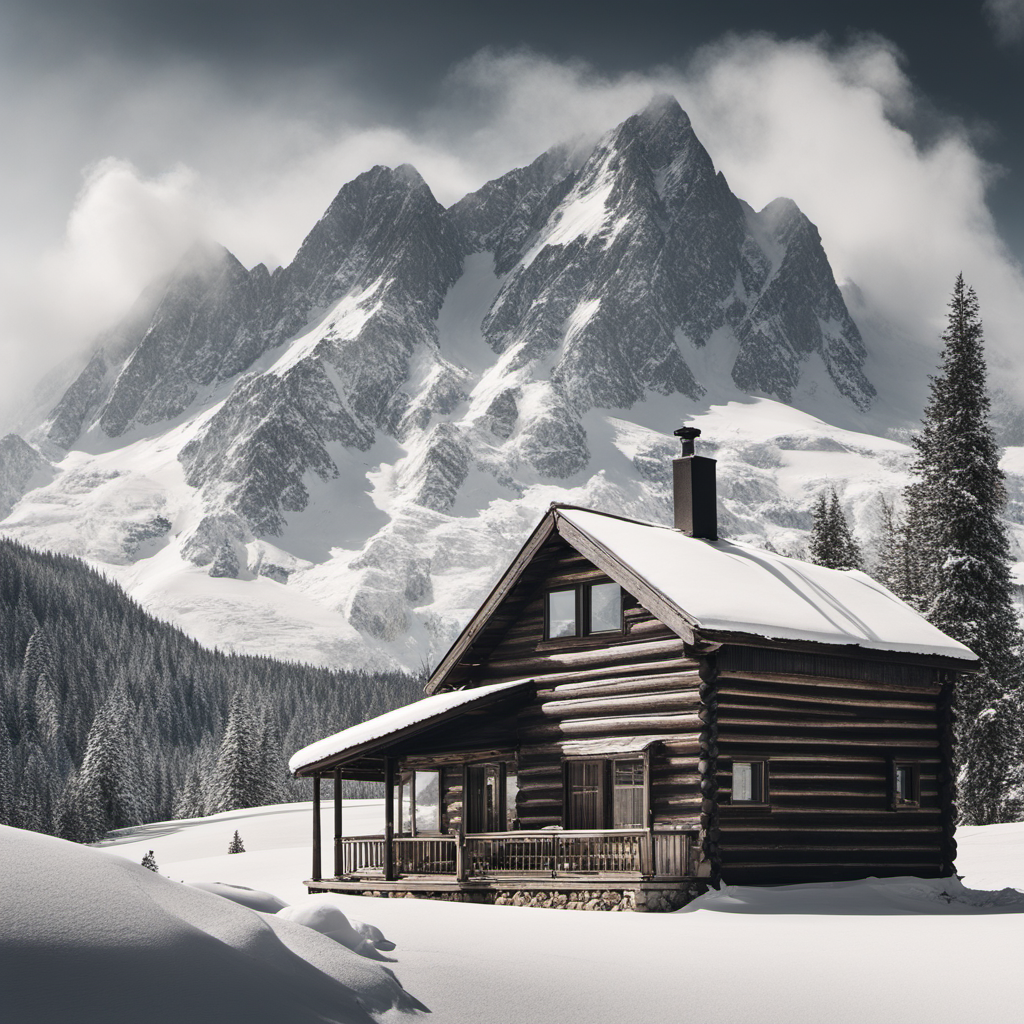
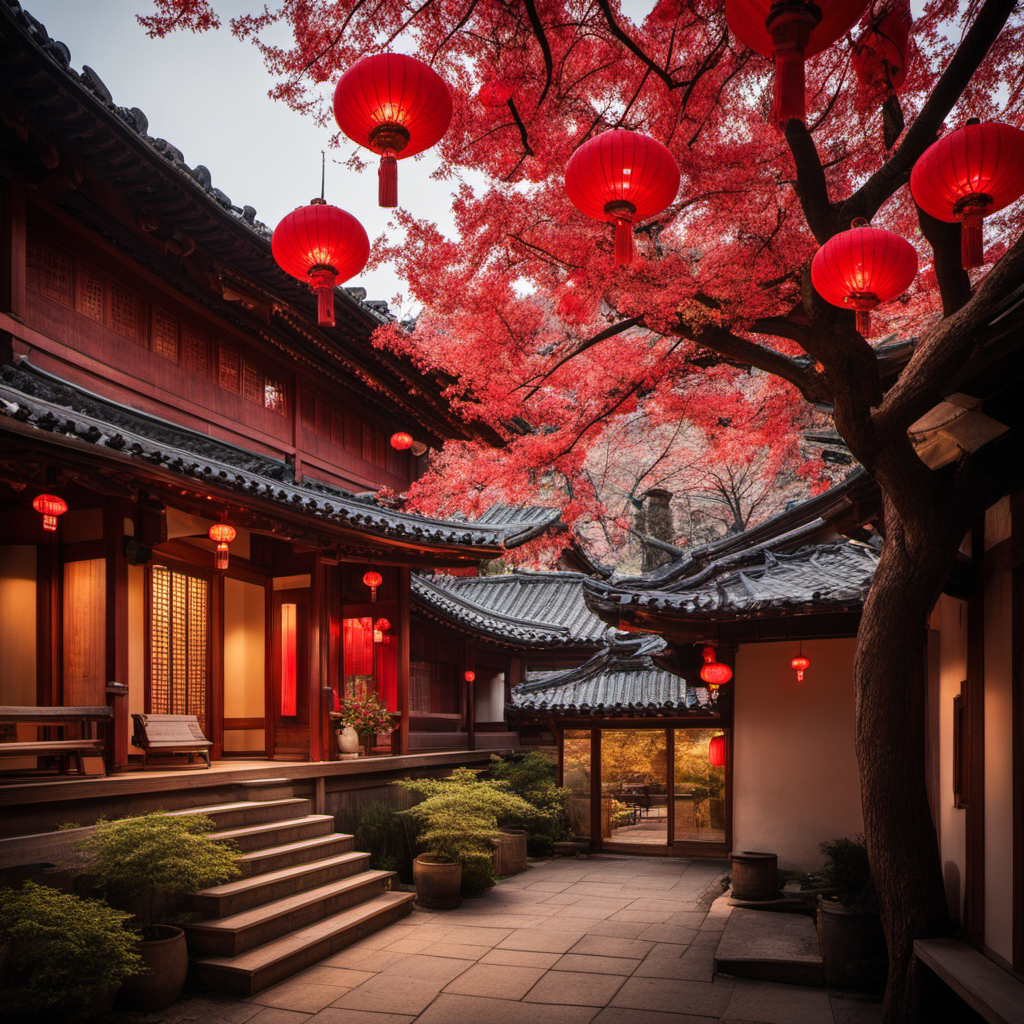
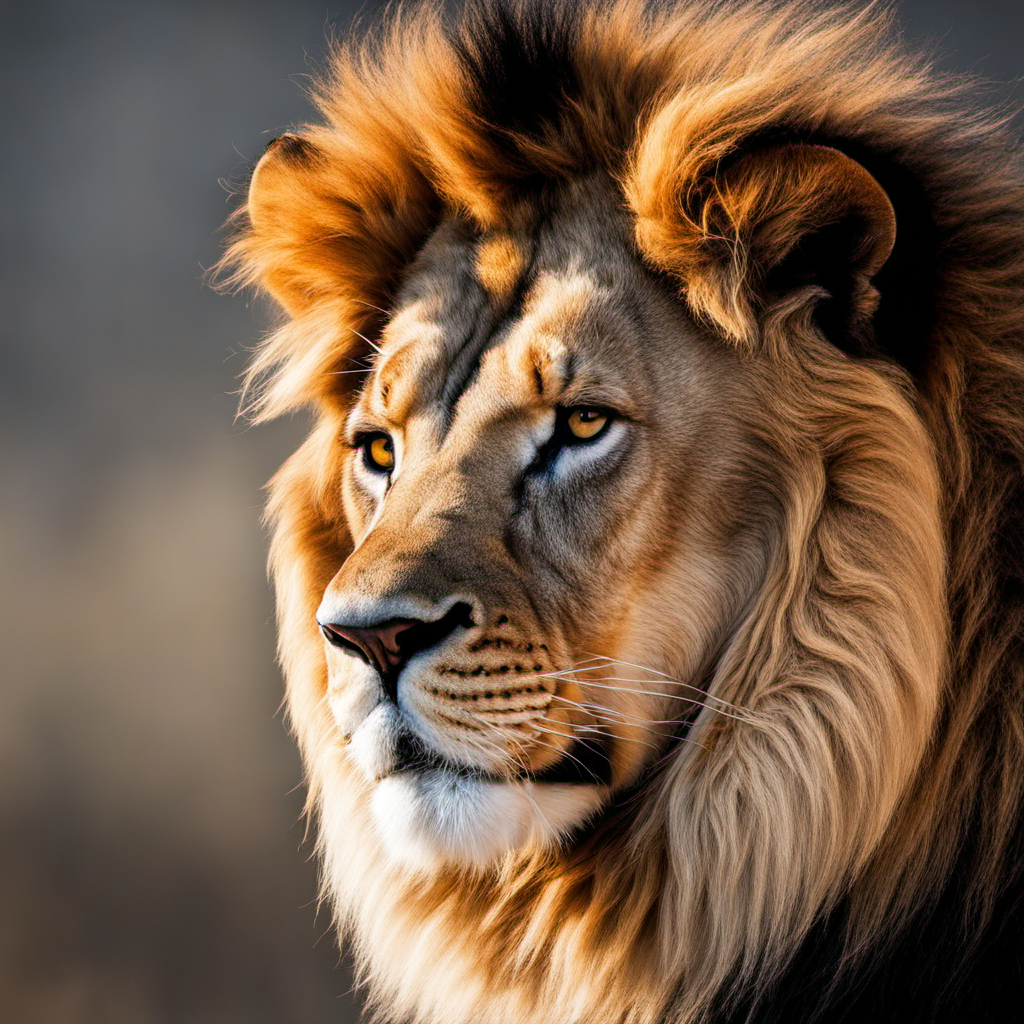
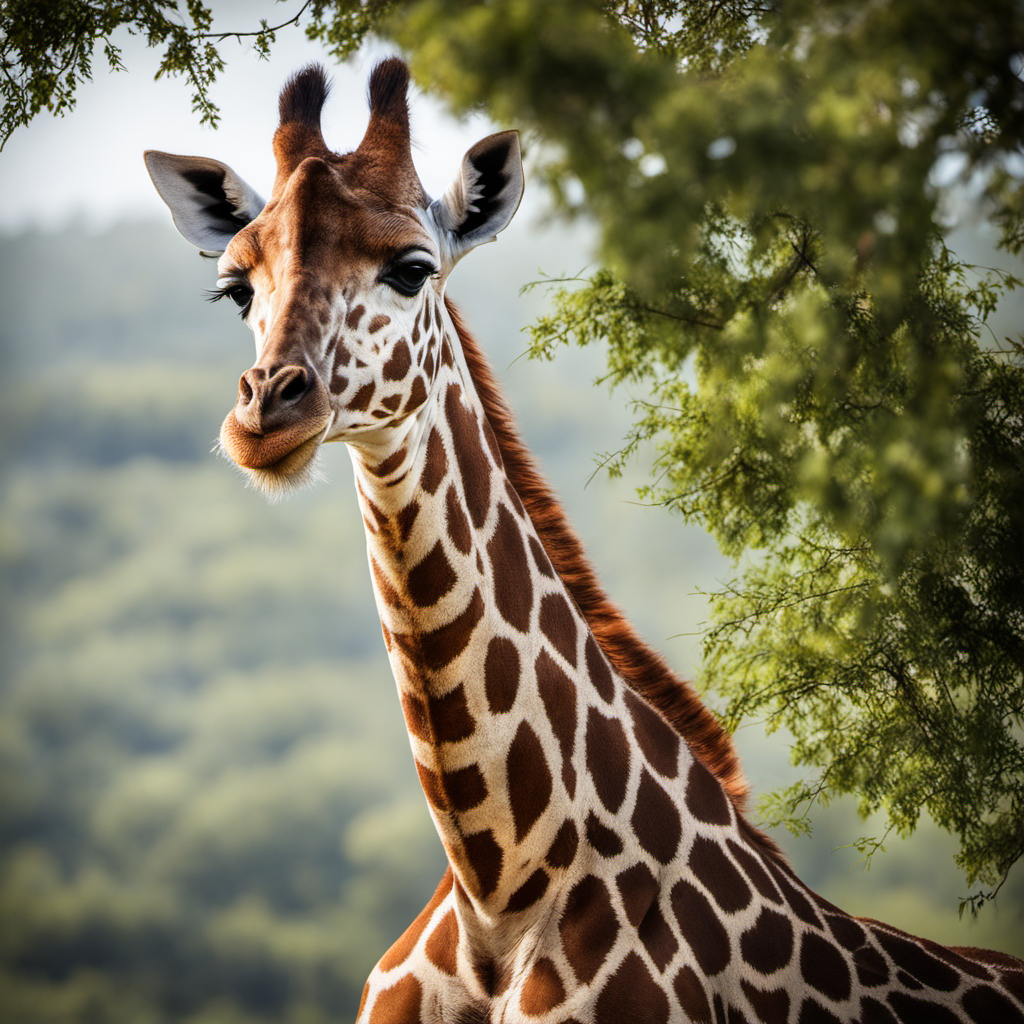
The rapid development of generative diffusion models has significantly advanced the field of style transfer. However, most current style transfer methods based on diffusion models typically involve a slow iterative optimization process, e.g., model fine-tuning and textual inversion of style concept. In this paper, we introduce FreeStyle, an innovative style transfer method built upon a pre-trained large diffusion model, requiring no further optimization. Besides, our method enables style transfer only through a text description of the desired style, eliminating the necessity of style images. Specifically, we propose a dual-stream encoder and single-stream decoder architecture, replacing the conventional U-Net in diffusion models. In the dual-stream encoder, two distinct branches take the content image and style text prompt as inputs, achieving content and style decoupling. In the decoder, we further modulate features from the dual streams based on a given content image and the corresponding style text prompt for precise style transfer.
We introduce FreeStyle, an innovative style transfer method built upon a pre-trained large diffusion model, requiring no further optimization.
@article{he2024freestyle,
title={Freestyle: Free lunch for text-guided style transfer using diffusion models},
author={He, Feihong and Li, Gang and Zhang, Mengyuan and Yan, Leilei and Si, Lingyu and Li, Fanzhang},
journal={arXiv preprint arXiv:2401.15636},
year={2024}
}